You have 1 article left to read this month before you need to register a free LeadDev.com account.
A calculated approach with clear phases and milestones is vital to successfully demonstrate the value Generative AI (GenAI) will bring to your organization.
Adoption should include a strategy that navigates technological challenges, aligns with business goals, and integrates smoothly with existing systems.
Here, YLD’s Head of Technology, shares how she has observed businesses adopting these tools to stay competitive, and what you can do to set a solid foundation for success.
GenAI is a long-term reality
Deloitte reports that 75% of organizations expect GenAI to impact their talent strategies within two years, with a primary focus on improving efficiency and productivity. Similarly, a 2023 report by MIT Technology Review Insights and Databricks highlights that companies are primarily deploying GenAI for customer experience, strategic analysis, and product innovation. AI adoption is also advancing in traditionally slower industries like manufacturing and the public sector.
Start by collaborating with other senior leaders or department heads in your organization to review your goals and objectives for the next few years, and pinpoint the areas of your business that require the most focus.
Here are some areas to consider for your initial GenAI deployment:
- Strategy: Improve decision-making by leveraging data-driven recommendations and predictive analytics to forecast customer behavior and other key metrics.
- Operations: Automate repetitive tasks to streamline workflows and reduce operational costs.
- Customer experience: Enhance service engagement with virtual assistants or chatbots that provide tailored responses based on individual customer data.
- Finance: Automate routine financial processes like expense reporting and reconciliation to increase accuracy and efficiency.
Here are other organizational areas where subsequent GenAI deployment can be beneficial:
- Business development: Increase competitive advantage by capturing market opportunities, optimizing pricing models, and enhancing product features.
- Product development: Assist in rapid prototyping and testing, enabling quicker iteration and refinement of product concepts based on stakeholder interviews, and other data collected during the product development lifecycle.
- IT security: Detect and respond to potential threats in real time using AI-driven systems to analyze network traffic, identify unusual patterns, and protect against data breaches and cyber-attacks.
- Human resources: Leverage GenAI for talent acquisition by automating resume screening and candidate matching based on skills and experience. Use AI-driven analytics to enhance employee engagement through personalized career development plans and to predict and address potential turnover risks.
- Marketing and sales: Boost performance by creating targeted marketing content, optimizing ad spend, and identifying high-potential leads.
Strengthen your data foundation
For senior engineering leaders, ensuring your organization maintains high-quality, accurate data is crucial for laying a strong foundation for AI initiatives.
While it’s important to aim for clean, unbiased data, it’s also essential to accept that no data will ever be completely perfect. Gaps like application failures and programming errors will always exist. Addressing these requires significant effort to clean and integrate data to effectively support large language models (LLMs).
To build a robust data foundation and confidently adopt new technologies, consider these strategies:
- Lambda architecture: Ideal for managing both live and historical data as it combines batch and real-time processing to maintain a consistent data infrastructure.
- Medallion architecture: Medallion architecture enhances data management, especially for reprocessing historical data with modern development techniques. This architecture uses multiple layers for data processing to ensure flexibility and accuracy:
- Bronze layer: Stores raw data.
- Silver layer: Filters, augments, and transforms data, allowing reprocessing with new filters or logic.
- Gold layer: Delivers cleaned-up results to enhance machine learning models.
- Archiving data: Organizations can easily accumulate terabytes or petabytes of data, requiring significant resources to query it quickly. To manage this data, it can be archived, deleted after a certain period, or transferred to an object store like S3. Consolidating daily and hourly files into weekly ones simplifies data management, improving access for teams and enabling faster results for engineers.
- Facilitate data interoperability: Standardizing data formats and using APIs make it easier to integrate systems and boost GenAI’s effectiveness throughout the business. Data interoperability is key for encouraging collaboration and smooth data exchange because it can access and share data across different systems.
- Robust data governance and monitoring: Assigning clear ownership for data elements is essential to ensure proper management, accountability, accuracy, security, and compliance. This accountability helps your business stay responsive as it scales.
Gradually deploy change
Start by introducing changes in specific departments, rather than implementing them across the entire organization at once. This phased approach allows your teams to adapt gradually, offering valuable feedback that helps refine the system before a broader rollout.
The following are real-world examples of how some of our clients have been leveraging GenAI, along with other AI technologies:
- Customer engagement
Automating and streamlining customer service is a quick win for businesses. A fintech client has been taking an iterative approach, using Retrieval-Augmented Generation (RAG) to enhance client-facing processes. By capturing and interpreting phone conversations, RAG extracts relevant information from a knowledge base, enabling customer service agents to provide more efficient customer support. In addition to summarizing conversations, RAG also performs sentiment analysis, which helps identify the overall tone of the calls. This allows for a deeper level of analysis for quality assurance.
RAG is beneficial for any organization with public-facing chat features. It not only accelerates response times but also supports human agents by delivering accurate answers from an up-to-date knowledge base, ensuring efficient handling of customer inquiries.
- Strategic decision-making
A broadcast media company has been focusing on reaching niche audience segments to expand their overall viewership. To achieve this, they replaced their legacy business intelligence APIs with a modern solution built on Snowflake to extract dynamic insights from their vast data in a performant and declarative manner. This boosted their data management capabilities by leveraging machine learning and GenAI to collect accurate, real-time data and feedback, which is then presented in a visually intuitive format.
- Customer insights and analytics
An energy supplier aimed to improve decision-making accuracy for buying wholesale energy by purchasing energy upfront in a day-ahead auction. With our support, they developed a machine learning model that challenges the industry standard algorithm “mean absolute percentage error” (MAPE), achieving a reduced error rate of 0.86%, compared to the industry average of 3-7%. This improvement resulted in risk savings worth £9 million.
Equip your team for success
Investing in data science and engineering expertise is crucial to keep up with the latest AI developments, but ensuring your team stays adaptable as the technology evolves is equally important.
Regular training sessions will keep everyone up to date. Extending this training beyond the tech department can also build confidence across the entire organization. Encourage hands-on experimentation and allow room for mistakes with GenAI technology.
Final thoughts
The more specific the information we collect, the better we can maximize GenAI’s benefits. Start by building a solid data foundation, identify clear use cases, and encourage curiosity and collaboration within and across teams.
Successful AI implementation is built on strong processes and high-quality data. For support with GenAI, data engineering, MLOps, data analysis, or data science, contact YLD.
Promoted Partner Content
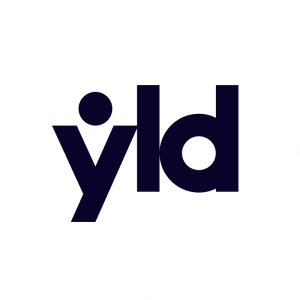